As part of a broader effort to analyze operational patterns in Montenegro’s healthcare sector, a predictive model was developed to estimate indicators related to the quality of healthcare services provided to citizens at the secondary and tertiary levels. The model was initially trained on a sample of 10,000 records and later expanded to over 40,000 records using the HPC cluster at UDG, which enabled the training process to complete in under one minute. All records were fully anonymized in accordance with ethical standards, and the dataset incorporated a wide range of temporal, demographic, and institutional variables relevant to healthcare access and service delivery patterns.
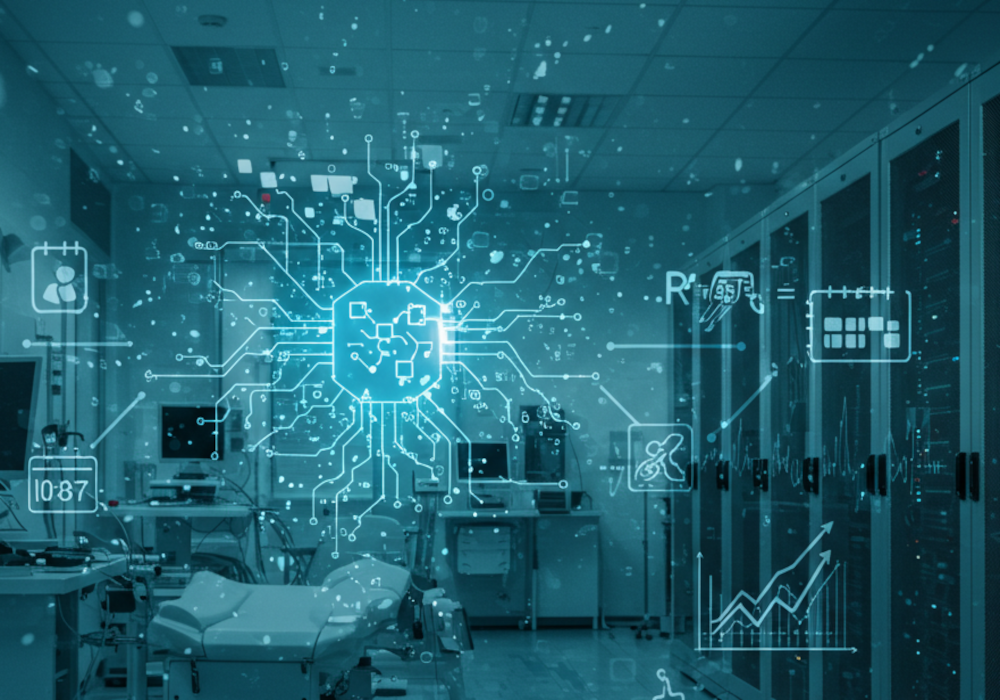
The model was implemented using the Random Forest Regressor algorithm, and additional experiments were conducted with alternative modeling techniques and target transformations. The baseline configuration achieved strong predictive performance (MAE ≈ 95 hours, R² = 0.87). While log-transformation and alternative algorithms such as XGBoost were evaluated, they did not yield improvements over the initial approach. Feature importance analysis revealed that factors such as month, weekday, and clinical unit had the highest impact on model outputs. Access to dedicated computing resources has been granted for further development, enabling continued model training and experimentation with advanced algorithms and optimization methods.
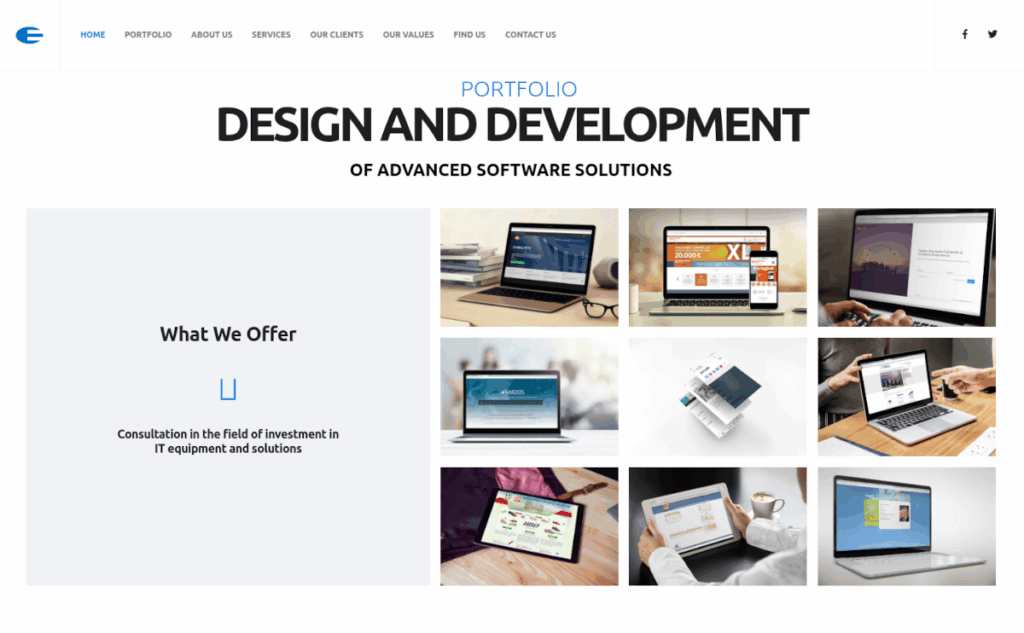