Ms. Tamara Pavlovic defended her MSc thesis on the use of HPC/AI for creating prediction models for breast cancer detection on 23.10.2024. With the support from NCC Montenegro, Ms Pavlovic did her research in the context of the HPC4S3ME project and the focus was on AI and computer vision applications in medicine. From the motivational point of view, we congratulate Tamara for finalizing and defending her thesis during the Breast Cancer Awareness Month (‘Pink October’) as people around the world adopt the pink colour and display a pink ribbon to raise awareness about breast health.
ABSTRACT – Artificial Intelligence (AI) is revolutionizing numerous sectors, including medicine, by offering innovative methods for diagnosing, treating, and researching diseases. This master’s thesis focuses on the application of AI in the diagnosis of breast cancer, using computer vision algorithms to analyze mammographic images. Through a combination of convolutional neural networks (CNNs) and deep learning, models have been developed that identify malignant changes, potentially contributing to earlier and more precise disease detection. The thesis examines in detail how AI can improve the efficiency of screening processes, reduce the time required for diagnosis, and enable a more personalized approach to treatment. In addition to technological progress, ethical issues such as patient safety and the transparency of AI systems are also considered. The results of this study confirm that the application of AI in breast cancer diagnostics can significantly enhance medical procedures. The models tested, ResNet152 and DenseNet121, demonstrated quite good performance in classifying breast cancer. Their AUC scores, which exceed the threshold of 0.9, indicate their potential for use in clinical practice. These findings not only contribute to the improvement of diagnostic processes but also open up opportunities for further research and development of AI technologies in medicine.
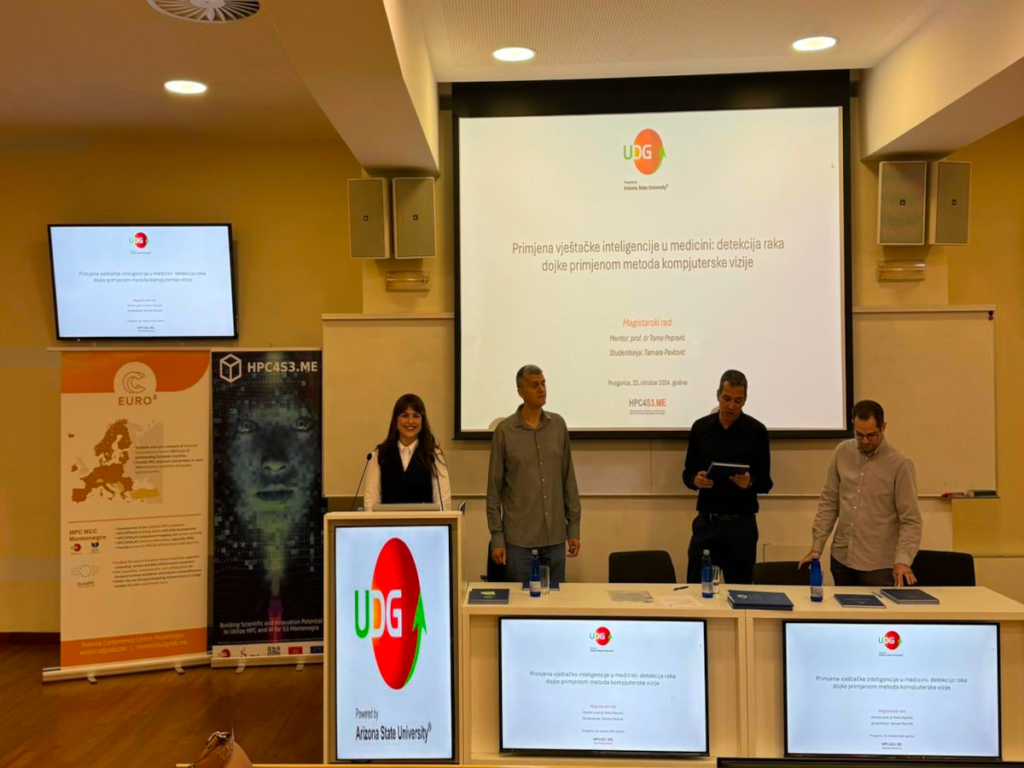
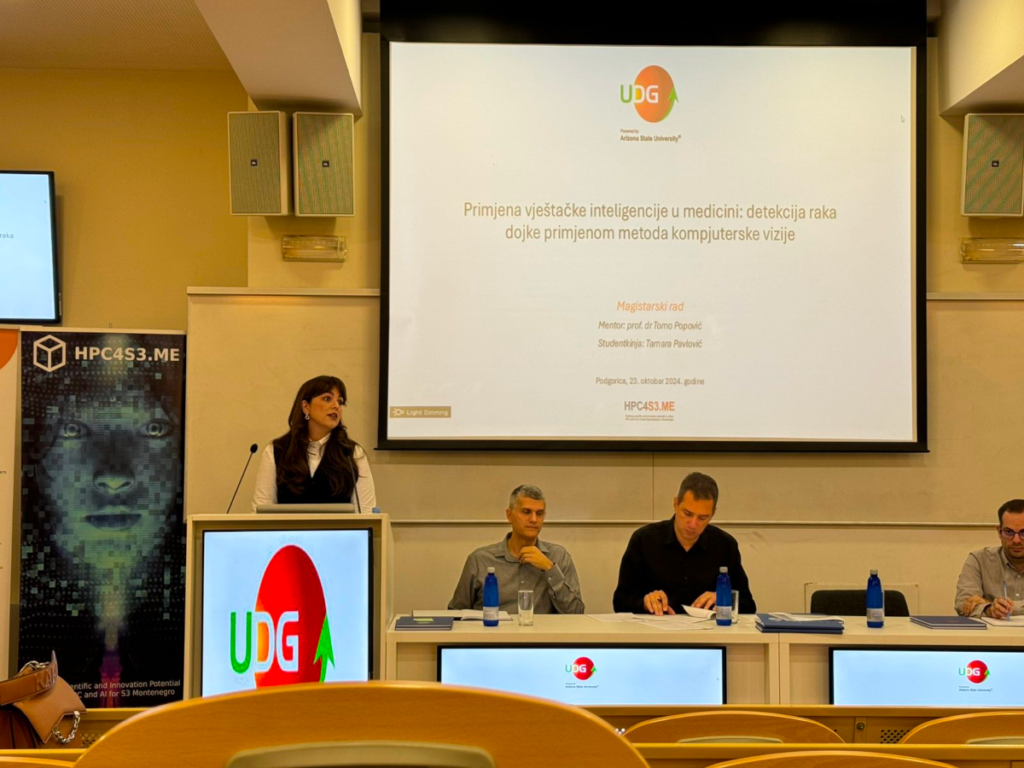