Breast Cancer Detection with Computer Vision and Image Processing
The analysis of mammographic images stands at the forefront of this challenge, a task that demands the discerning eye of experienced radiologists to identify the slightest hints of malignancy. These professionals navigate through a vast sea of mammograms, each potentially harboring subtle indicators of early-stage cancer that are easily missed amidst the complexity of human tissue patterns. The dual challenges of maintaining detection accuracy amidst a high volume of intricate images, and the variability introduced by radiologist fatigue, present significant barriers to timely and accurate diagnosis.
The traditional reliance on subjective interpretation compounds these challenges, introducing delays and potential inconsistencies in patient care. The diagnostic landscape called for an innovative solution that could augment human expertise with the precision and efficiency of technology, particularly in harnessing the power of object detection models within Machine Learning (ML). The introduction of ML object detection models promised a revolutionary approach to identifying abnormalities, yet integrating these advanced algorithms with the nuanced realm of mammography posed its own set of intricate challenges. It demanded not only a leap in computational capability but a new paradigm in the interpretation and processing of mammographic imagery.
SOLUTION:
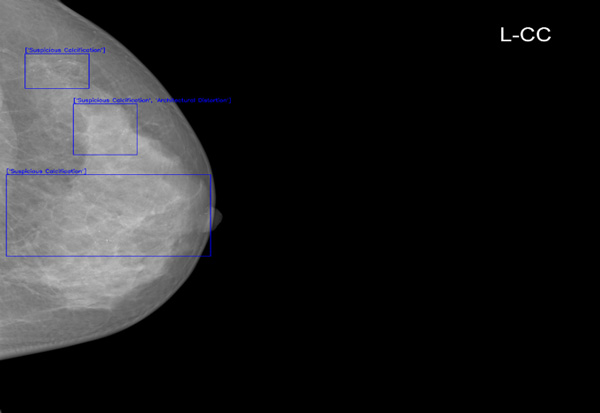

The journey to enhance mammographic analysis leveraged the convergence of digital image processing and Machine Learning (ML) to unlock new dimensions in breast cancer detection. State-of-the-art image processing techniques, like Contrast Limited Adaptive Histogram Equalization (CLAHE), not only elevated the visualization quality by 40% but also laid the groundwork for sophisticated ML object detection models to analyze these images with unprecedented precision. This strategic integration facilitated the use of ML models capable of identifying a spectrum of abnormalities. The deployment of object detection models within this framework exemplifies the forward leap in automating the diagnostic process, aiming to complement radiologists’ expertise by providing them with AI-augmented insights.
Through the collaborative spirit of the University of Montenegro and the University of Donja Gorica, this initiative has pushed the envelope of mammographic evaluation. Supported by EuroCC, this project harnessed advanced computational power, improving the efficiency of image analysis by 25% and significantly reducing manual examination time by 35%.
This synergy has not only optimized the diagnostic workflow but also addressed the challenge of processing vast mammographic datasets within the constraints of available GPU resources, demonstrating the potential of HPC to bridge the gap.
Through the meticulous application of image enhancement methods and the strategic use of ML object detection models, the project has pioneered a pathway towards more accurate, efficient, and comprehensive breast cancer screening, marking a significant stride towards the modernization of health diagnostics.
BENEFITS:
The application of AI/ML and HPC in this context has brought multiple benefits:
- Significantly faster detection of abnormalities, leading to earlier diagnosis and increasing the chances for successful treatment.
- Enhanced precision in identifying different types of tumors, enabling personalized treatment approaches.
- Reduced time required for image analysis, freeing radiologists for other critical tasks.
- Potential reduction in patient stress and anxiety thanks to faster analysis results.
- The project represents an important step towards the modernization and digitalization of health diagnostics, highlighting the importance of integrating the latest technological advancements into medical processes.